Research
AI-Assisted Programming
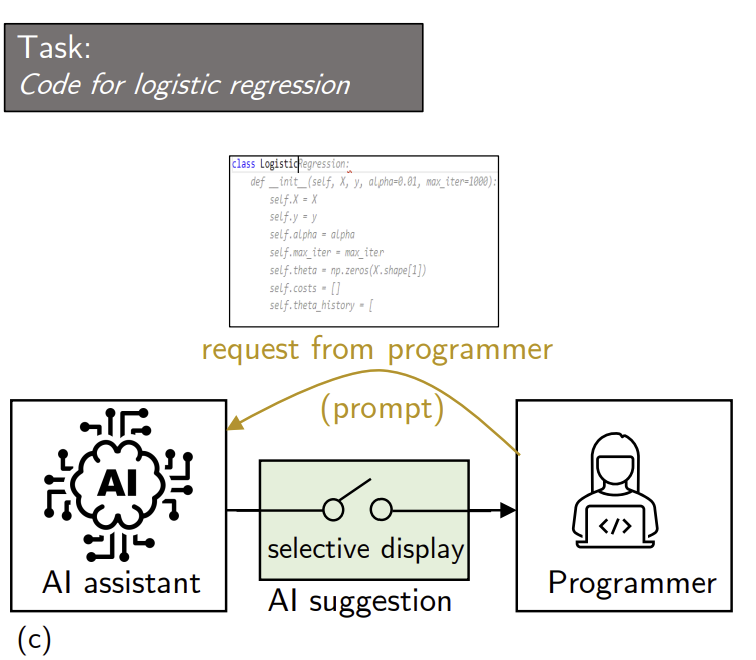
AI has changed the way we write code for a lot of us. LLMs have been adopted by millions of programmers to help them write their code. The forms of AI-assisted programming are diverse, ranging from code completions (original GitHub Copilot), chat-based general-purpose AI assistants (ChatGPT), and newer forms that integrate AI agents and code edits. The goal of my research is to understand how people interact with these AI systems, how we can evaluate their impact on programmers' performance, and how we can design new interfaces that integrate AI.
My previous work includes:
Learning to Defer to Humans
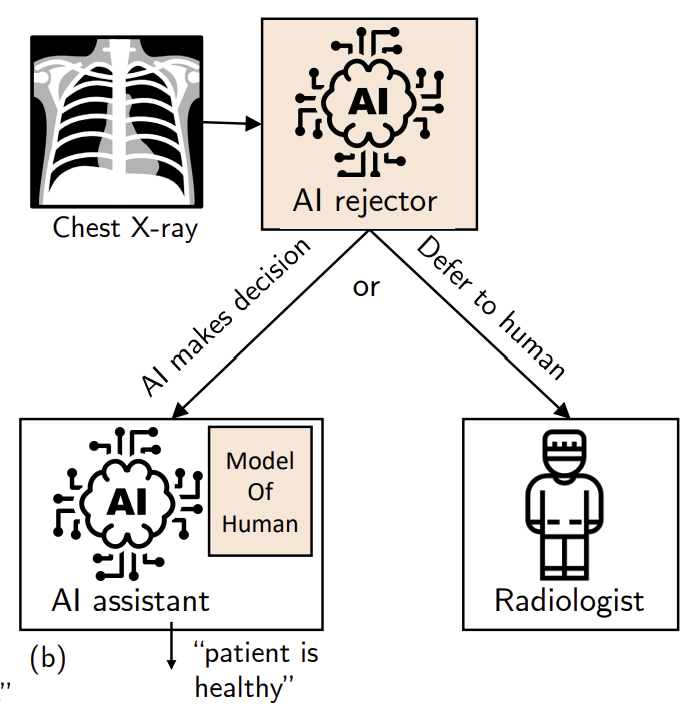
How do we combine AI systems and human decision-makers to both reduce error and alleviate the burden on the human? AI systems are starting to be frequently used in combination with human decision-makers, including in high-stakes settings like healthcare and content moderation. A possible way to combine the human and the AI is to learn a 'rejector' that queries either the human or the AI to predict on each input. This allows us to route examples to the AI model, where it outperforms the human, so as to simultaneously reduce error and human effort. Moreover, this formulation allows us to jointly optimize the AI so as to complement the human’s weaknesses, and to optimize the rejector to allow the AI to defer when it is unable to predict well. This problem is referred to as the 'learning to defer' problem.
My previous work includes:
An article on this work can be found
here.
Teaching Humans How to Interact with AI
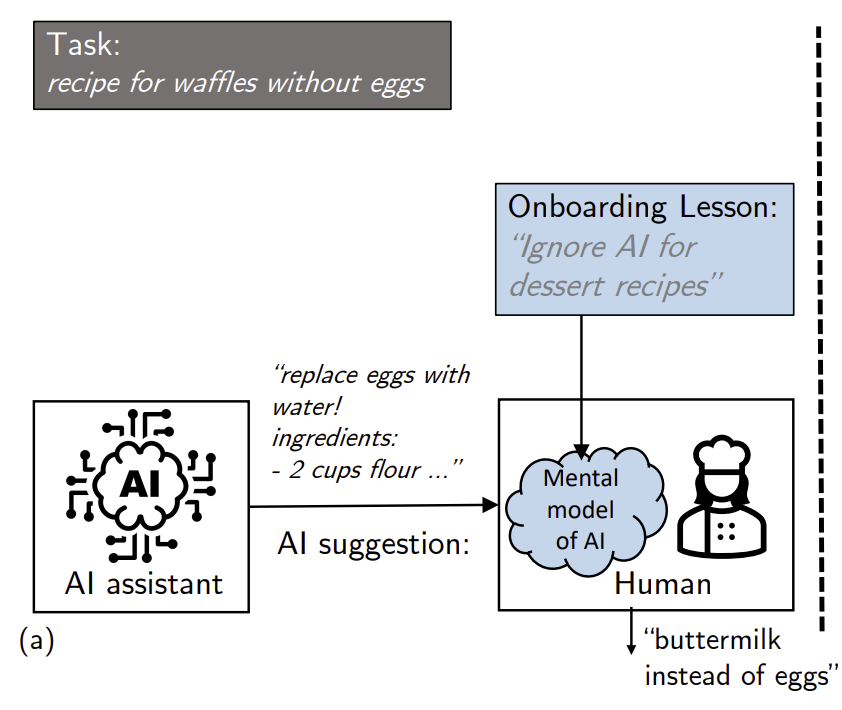
A key question is how does the human expert know when to rely on the AI for advice. The literature on human-AI collaboration has often revealed that humans often underperform expectations when working with AI systems. The negative results of human-AI performance may be attributed to a few possible reasons. First, humans can have miscalibrated expectations about AI’s ability, which leads to over-reliance. Second, the cost of verifying the AI’s answer with explanations might be too high thus providing a bad cost-benefit tradeoff for the human and leading to either over-reliance or under-reliance on the AI. Finally, the AI explanations do not enable the human to verify the correctness of the AI’s answer and thus are not as useful for human-AI collaboration.
We make the case for the need to initially onboard the human decision-maker on when and when not to rely on the automated agent. We propose that before an AI agent is deployed to assist a human decision-maker, the human is taught through a tailored onboarding phase how to make decisions with the help of the AI. The purpose of the onboarding is to help the human understand when to trust the AI and how the AI can complement their abilities.
My previous work includes:
An article on this work can be found
here.
You can find my full list of publications on my Google Scholar